Non-Parametric and Semi-Parametric Methods in Econometrics: A Deep Dive
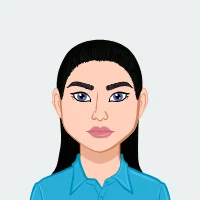
Navigating the intricate landscape of econometrics, non-parametric and semi-parametric methods emerge as indispensable tools for discerning patterns in economic data. As an expert guiding students through their econometrics assignments, I elucidate the nuanced realm of non-parametric techniques, which eschew stringent assumptions about the functional form of relationships. Kernel density estimation, local polynomial regression, and spline methods empower scholars to unveil intricate structures within data, particularly useful when facing nonlinear relationships. If you need assistance with your econometrics assignment, I'm here to provide expert support and guidance to ensure your success in your studies.
In parallel, semi-parametric models seamlessly blend parametric and non-parametric components, offering a flexible compromise. This hybrid approach accommodates both predefined structural parameters and the freedom to capture intricate patterns without imposing excessive assumptions. I guide students in mastering methodologies such as partially linear models and generalized additive models, fostering a comprehensive understanding of their application in diverse economic contexts.

Moreover, our exploration extends to the inherent challenges and caveats of these methods, instilling a discerning mindset in students. Harnessing these techniques requires not only technical proficiency but also a keen appreciation for the data's underlying dynamics. As we delve into the intricacies of non-parametric and semi-parametric econometrics, students are equipped not only to complete assignments but to contribute to the evolving landscape of economic analysis.
Unveiling the Intricacies of Non-Parametric Econometric Techniques: A Comprehensive Analysis
In the realm of econometrics, where precision and accuracy are paramount, non-parametric techniques have emerged as powerful tools for unraveling complex relationships in economic data. As an expert guiding students through the intricacies of econometrics assignments, it becomes imperative to delve into the world of non-parametric methods. This comprehensive analysis aims to shed light on the nuances of non-parametric econometric techniques, providing a roadmap for students to navigate and master these sophisticated tools.
Understanding Non-Parametric Techniques:
Non-parametric techniques, unlike their parametric counterparts, do not rely on specific functional forms for underlying relationships. Instead, they offer flexibility in modeling by allowing data-driven patterns to guide the analysis. This flexibility is particularly valuable when the true functional form of the relationship is unknown or complex, making non-parametric techniques an indispensable asset in econometrics.
Kernel Density Estimation:
One of the fundamental non-parametric techniques is Kernel Density Estimation (KDE). In the world of econometrics assignments, KDE is often employed to estimate the probability density function of a random variable. The method involves placing a kernel (a smooth, symmetric function) at each data point and summing their contributions to create a continuous density estimate. This technique proves invaluable in visualizing and understanding the distribution of economic variables, aiding students in exploring data patterns beyond traditional parametric assumptions.
Local Polynomial Regression:
Local Polynomial Regression, another non-parametric gem, allows for the estimation of regression relationships without imposing a global functional form. By fitting polynomials to local subsets of the data, this technique captures varying degrees of complexity in different regions, offering a nuanced perspective on the data structure. For students grappling with assignments that involve non-linear relationships, local polynomial regression provides a powerful alternative to rigid parametric specifications.
Kernel Regression:
Kernel Regression extends the principles of KDE to estimate conditional expectations in regression models. As an expert guiding students, emphasizing the application of kernel regression proves beneficial in scenarios where the relationship between variables is nonlinear and lacks a predetermined form. By providing a flexible framework, kernel regression enables students to adapt to the intricacies of diverse economic datasets.
Advantages and Challenges:
Non-parametric techniques offer several advantages, such as increased flexibility and the ability to model complex relationships. However, they also present challenges, including the potential for overfitting and the need for careful consideration of tuning parameters. Guiding students through these nuances is crucial, instilling a balance between flexibility and caution in the application of non-parametric methods.
Applications in Economic Research:
As students engage with econometrics assignments, highlighting real-world applications of non-parametric techniques is essential. These methods find utility in diverse economic research areas, including labor economics, finance, and environmental economics. Demonstrating the relevance of non-parametric approaches instills a deeper understanding of their practical implications, preparing students for the dynamic challenges of applied econometrics.
In the ever-evolving landscape of econometrics, non-parametric techniques stand as indispensable tools for analyzing intricate economic relationships. As an expert guiding students through their assignments, unraveling the complexities of Kernel Density Estimation, Local Polynomial Regression, and Kernel Regression is paramount. By imparting a comprehensive understanding of non-parametric econometric techniques, students can navigate the intricacies of economic data with confidence, ultimately contributing to the advancement of empirical research in the field.
Exploring the Foundations: Understanding the Basics of Non-Parametric Techniques
In the realm of econometrics, students often navigate the intricate terrain of statistical analysis to unveil economic patterns and relationships. Delving into this intricate landscape, understanding the basics of non-parametric techniques becomes paramount. As an expert guiding students through their econometrics assignments, I emphasize the foundational significance of non-parametric methods.
Non-parametric techniques, unlike their parametric counterparts, make minimal assumptions about the underlying data distribution. In the world of econometrics, this flexibility is crucial when dealing with real-world data that may not conform to strict statistical assumptions. From kernel density estimation to the Wilcoxon rank-sum test, non-parametric tools provide robust alternatives for students grappling with diverse datasets.
My guidance encourages students to appreciate the simplicity and versatility of non-parametric techniques. Whether deciphering the intricacies of the Mann-Whitney U test or comprehending the elegance of the Kolmogorov-Smirnov statistic, these foundational skills empower students to extract meaningful insights from empirical data.
Furthermore, I stress the importance of understanding when to employ non-parametric methods, elucidating their advantages in scenarios where assumptions of parametric models might falter. This nuanced comprehension fosters a holistic approach to econometrics assignments, equipping students to choose and apply the most appropriate tools for a given research question. In essence, exploring the foundations of non-parametric techniques not only enhances statistical acumen but also equips students with a versatile toolkit essential for unraveling the complexities of economic phenomena.
Navigating the Complex Landscape: Exploring Applications, Limitations, and Innovations
In the ever-evolving realm of economics, econometrics stands as a crucial tool, weaving together theory and data to provide insights into complex economic phenomena. As an expert guiding students through the intricate landscape of econometrics assignments, I find myself at the intersection of theory, statistics, and real-world applications. In this discourse, we will delve into the multifaceted nature of econometrics, unraveling its applications, acknowledging its limitations, and exploring the latest innovations that shape the field.
Econometrics is the bridge that connects economic theories with empirical evidence, allowing economists to test hypotheses and draw meaningful conclusions. Its applications are vast and varied, ranging from macroeconomic forecasting to microeconomic policy analysis. When guiding students, I emphasize the importance of understanding the context within which econometric tools are applied. Whether estimating the impact of fiscal policies on GDP or unraveling the intricacies of consumer behavior, econometrics equips students with the analytical prowess needed to navigate the complexities of economic phenomena.
One primary application of econometrics lies in causal inference, where researchers seek to establish cause-and-effect relationships between economic variables. As students grapple with assignments, they must learn to identify and overcome the challenges inherent in distinguishing correlation from causation. This involves employing advanced statistical techniques, such as instrumental variables or difference-in-differences, to address endogeneity and confounding issues. By guiding students through these applications, I empower them to critically evaluate economic relationships and contribute to the advancement of economic knowledge.
However, as with any tool, econometrics has its limitations. Assumptions of linearity, homoscedasticity, and independence of errors, among others, pose challenges that students must be aware of and address in their assignments. I guide students to develop a keen understanding of these assumptions, encouraging them to assess the robustness of their models and interpret results cautiously. Acknowledging these limitations is a crucial step in fostering a nuanced perspective on the capabilities and boundaries of econometrics.
In the dynamic landscape of econometrics, innovations continually shape the field, providing students with exciting avenues for exploration. Advanced techniques such as machine learning and Bayesian econometrics are gaining prominence, offering novel ways to model complex relationships and uncertainties. I guide students to stay abreast of these innovations, fostering a spirit of curiosity and adaptability. By incorporating the latest advancements into their assignments, students not only showcase their analytical skills but also contribute to the evolution of econometric methodologies.
Moreover, the increasing availability of big data and computational power has revolutionized econometric research. I encourage students to harness these resources, emphasizing the importance of incorporating real-world data and leveraging cutting-edge computational tools in their assignments. By doing so, they not only enhance the relevance of their work but also gain valuable skills that are highly sought after in today's data-driven economy.
As an expert guiding students through their econometrics assignments, I navigate the complex landscape of applications, limitations, and innovations in this vital field. By instilling a deep understanding of econometric principles, encouraging critical thinking, and promoting the integration of the latest innovations, I empower students to embark on a journey of discovery within the ever-evolving world of econometrics. In doing so, they not only excel in their assignments but also contribute to the ongoing advancement of economic knowledge and analysis.
The Hybrid Realm: Demystifying Semi-Parametric Econometrics and its Role in Empirical Research
In the ever-evolving landscape of empirical research, econometrics stands as the bedrock that bridges economic theory with real-world data. Within this intricate realm, the emergence of semi-parametric econometrics has garnered significant attention, offering a nuanced approach to modeling complex relationships that traditional parametric methods struggle to capture. As an expert guiding students through the labyrinth of econometrics assignments, it becomes imperative to demystify the intricacies of semi-parametric techniques and illuminate their crucial role in enhancing the empirical rigor of economic studies.
At its core, semi-parametric econometrics strikes a delicate balance between the flexibility of non-parametric methods and the efficiency of parametric models. In the conventional parametric framework, assumptions about the functional form of the relationship between variables are made, often resulting in models that may not adequately reflect the underlying data-generating process. Semi-parametric methods, however, allow for a more adaptive approach, enabling the data to dictate the functional form while still incorporating parametric elements.
One of the key advantages of semi-parametric models lies in their ability to handle situations where the true relationship between variables is complex and nonlinear. This is particularly valuable in economics, where relationships are seldom linear and often exhibit intricate patterns. By avoiding rigid assumptions about functional forms, semi-parametric techniques provide a more accurate representation of the underlying economic reality, capturing nuances that may be overlooked by traditional models.
A cornerstone of semi-parametric econometrics is the kernel smoothing method. This technique allows for a flexible estimation of the underlying probability density function, enabling researchers to uncover patterns in the data without imposing restrictive parametric assumptions. As students navigate through their econometrics assignments, understanding the intricacies of kernel smoothing becomes paramount in unlocking the potential of semi-parametric methods.
Moreover, semi-parametric models offer a robust solution to the pervasive issue of omitted variable bias. In empirical research, omitting relevant variables can lead to biased parameter estimates and flawed conclusions. Semi-parametric techniques, by virtue of their adaptability, are better equipped to handle omitted variable bias, offering a more accurate depiction of the relationships within the data.
In guiding students through the realm of semi-parametric econometrics, it is crucial to emphasize the complementarity of these methods with traditional parametric approaches. Semi-parametric models, when integrated judiciously with parametric specifications, provide a comprehensive toolkit for researchers. This synergy allows for a more holistic understanding of the data, harnessing the strengths of both paradigms while mitigating their respective weaknesses.
However, it is essential to underscore the challenges that come with employing semi-parametric methods. The increased flexibility comes at the cost of potential model complexity and computational demands. As an expert mentor, guiding students through the intricacies of model selection and validation becomes imperative to ensure the judicious application of these advanced techniques.
The hybrid realm of semi-parametric econometrics represents a paradigm shift in empirical research, offering a nuanced and adaptive approach to modeling complex economic relationships. As an expert guiding students through their econometrics assignments, demystifying the intricacies of semi-parametric techniques and emphasizing their pivotal role in enhancing empirical rigor becomes not only a pedagogical responsibility but a commitment to equipping the next generation of economists with the tools necessary to navigate the evolving landscape of economic research.
Striking a Balance: Unveiling the Power and Flexibility of Semi-Parametric Models
Navigating the intricate landscape of econometrics, students often grapple with the delicate balance between model simplicity and capturing the complexities inherent in real-world data. As an expert guiding them through the labyrinth of statistical analysis, I advocate for the nuanced integration of semi-parametric models—a powerful tool in the econometrician's arsenal.
Semi-parametric models offer a middle ground, marrying the flexibility of non-parametric methods with the efficiency of parametric models. In econometrics assignments, I emphasize the pivotal role these models play in accommodating diverse data structures and capturing latent patterns without succumbing to overfitting. Striking a balance is paramount, and semi-parametric models provide the means to navigate this equilibrium delicately.
By allowing for a mix of parametric and non-parametric components, students can address both linearity assumptions and capture non-linear relationships inherent in economic phenomena. Whether tackling time-series data or examining intricate causal relationships, semi-parametric models grant the versatility needed to discern underlying trends without sacrificing interpretability.
In the realm of econometrics, embracing the power and flexibility of semi-parametric models unveils a sophisticated approach to modeling that empowers students to extract meaningful insights from data while navigating the complexities of real-world economic phenomena. It's not just about equations and coefficients; it's about understanding the intricate dance between simplicity and capturing the richness of economic reality.
Beyond the Basics: Advanced Techniques and Emerging Frontiers in Non-Parametric and Semi-Parametric Econometric Research
While a solid understanding of foundational concepts is crucial, the ever-evolving field of econometrics demands a deeper exploration into advanced techniques and emerging frontiers. In this discourse, we will delve into the realm of non-parametric and semi-parametric econometric research, unlocking the doors to a world of sophisticated methodologies that can elevate students' analyses to new heights.
Non-parametric econometrics breaks away from the constraints of traditional linear models, allowing researchers to explore relationships without rigid assumptions about the functional form. Kernel density estimation and non-parametric regression are powerful tools in this arsenal. These techniques enable students to capture complex patterns in the data, offering a more nuanced understanding of economic phenomena.
Consider, for instance, a scenario where the relationship between two variables is highly nonlinear. A non-parametric approach can flexibly model this intricate relationship without imposing predetermined shapes, providing a more accurate depiction of the underlying dynamics. Students are encouraged to experiment with various kernel functions and bandwidths, a process akin to tuning an instrument for optimal performance.
Moving further into the advanced terrain, semi-parametric econometrics strikes a delicate balance between non-parametric flexibility and the efficiency of parametric models. Here, students can integrate the strengths of both worlds, leveraging the flexibility of non-parametric methods in conjunction with the precision of parametric specifications.
Local polynomial regression, a cornerstone of semi-parametric analysis, allows students to adapt the level of smoothing to different regions of the data. This adaptability proves invaluable in capturing intricate patterns while avoiding overfitting. As a guide, I often emphasize the importance of understanding the trade-off between bias and variance inherent in these techniques, encouraging students to strike an optimal balance that aligns with the specific characteristics of their datasets.
Emerging frontiers in econometric research present exciting opportunities for students to contribute to the ever-expanding knowledge base. Machine learning techniques, such as ensemble methods and deep learning, are increasingly finding applications in econometrics. By harnessing the power of artificial intelligence, students can navigate vast datasets and uncover hidden relationships, pushing the boundaries of what was once deemed possible in econometric analysis.
The fusion of econometrics with cutting-edge technologies also extends to Bayesian non-parametrics, where students can explore the intricacies of modeling uncertainty using Bayesian methods without rigid parametric assumptions. This approach opens doors to a richer understanding of uncertainty and variability in economic relationships.
In guiding students through these advanced techniques, it is imperative to foster a mindset of curiosity and a commitment to continuous learning. Practical applications, real-world examples, and hands-on projects can transform abstract concepts into tangible skills. Moreover, emphasizing the importance of robustness checks and sensitivity analyses becomes paramount as students navigate the complexities of advanced econometric methodologies.
Beyond the basics lie a plethora of advanced techniques and emerging frontiers in non-parametric and semi-parametric econometric research. As an expert guiding students, my role extends beyond imparting knowledge to nurturing a mindset that embraces innovation and embraces the evolving nature of econometrics. By delving into these advanced realms, students not only enhance their analytical skills but also contribute to the dynamic and ever-expanding landscape of econometric research.
Conclusion
In conclusion, the exploration of non-parametric and semi-parametric methods in econometrics provides a nuanced understanding of statistical techniques that go beyond the conventional parametric frameworks. As an expert guiding students through their econometrics assignments, it is evident that these methods offer a valuable toolbox for analyzing complex economic relationships that may not conform to strict parametric assumptions.
Non-parametric methods, with their flexibility and ability to capture intricate patterns in data, empower students to model relationships without imposing rigid functional forms. The kernel density estimation, non-parametric regression, and bootstrapping techniques enhance the toolkit, allowing for robust analysis in scenarios where assumptions about the underlying data distribution are uncertain.
On the other hand, semi-parametric methods strike a balance between flexibility and structure. By incorporating both parametric and non-parametric components, students gain the advantage of capturing the broad trends while still accounting for specific features in the data. Methods such as the partially linear models and spline-based approaches contribute to a more comprehensive analysis, particularly when facing situations where fully parametric models fall short.
In guiding students, it is crucial to emphasize the importance of understanding the underlying assumptions and limitations of each method. Non-parametric and semi-parametric techniques, while powerful, require a thoughtful approach to ensure accurate and reliable results. Additionally, hands-on experience with real-world data is essential for honing the skills needed to apply these methods effectively.
Moreover, the deep dive into non-parametric and semi-parametric methods illuminates the evolving landscape of econometrics, showcasing its adaptability to the complexities of economic phenomena. The expert guidance provided to students extends beyond mere completion of assignments; it cultivates a deeper appreciation for the intricacies of econometric analysis and equips them with tools to tackle real-world challenges in economic research.
In conclusion, as an expert guiding students through the exploration of non-parametric and semi-parametric methods in econometrics, fostering a balance between theoretical understanding and practical application is paramount. By embracing these advanced techniques, students can elevate their analytical skills, contributing to a more nuanced and sophisticated approach to economic modeling and analysis.