Claim Your Offer
Get 10% off on all Economics assignments at www.economicsassignmenthelp.com! Whether it's Microeconomics, Macroeconomics, or any other topic, save big while getting expert help. Our experienced experts ensure top-quality solutions tailored to your needs. Don't miss out—grab this offer today and ace your Economics assignments with confidence!
We Accept
Customized Regression Analysis Assignment Help at Budget-Friendly Rates
At EconomicsAssignmentHelp.com, we understand the importance of affordability without compromising quality. That's why we offer customized Regression Analysis Assignment Help at budget-friendly rates. Our team of regression analysis assignment experts is dedicated to providing tailored assistance to meet your needs without straining your wallet. Whether you're a student seeking help with regression analysis assignments or guidance on statistical modeling, we're here to help. Trust us for top-notch support that fits your budget and helps you excel academically.
Service | Price Range |
---|---|
Basic Regression Analysis Tasks | $15 - $30 |
Intermediate Regression Analysis Assignments | $35 - $60 |
Advanced Regression Analysis Techniques | $65 - $100 |
Comprehensive Regression Analysis Projects | $120 - $200+ |
Expedited Service (Any Level) | Additional 50% |
Consultation & Workflow Optimization | $20 - $50 Per Hour |
- The Increasing Demand for Online Regression Analysis Assignment Help
- A Winning Choice: The Benefits of Opting for Our Regression Analysis Assignment Help
- How to Get Our Regression Analysis Assignment Help Service?
- Our Regression Analysis Assignment Help Service Covering Multiple Topics
The Increasing Demand for Online Regression Analysis Assignment Help
As the complexity of Regression Analysis assignments continues to rise, the demand for online Regression Analysis assignment help is experiencing a significant upsurge. Students seek help with Regression Analysis assignments to navigate through intricate statistical models and interpret results accurately. At EconomicsAssignmentHelp.com, our team of Regression Analysis assignment experts is equipped to provide tailored assistance, ensuring students grasp key concepts and excel in their coursework.
With the convenience of online platforms, students can access expert guidance from anywhere, empowering them to tackle challenging assignments with confidence. If you are wondering who will do my Regression Analysis assignment? Trust us for top-notch economics assignment help service that elevates your understanding and academic performance.
- Complexity of Assignments: Regression Analysis assignments often involve intricate statistical models and data analysis techniques, which can be challenging for students to comprehend and apply effectively without expert guidance.
- Need for Interpretation: Understanding regression output, interpreting coefficients, assessing model fit, and drawing meaningful conclusions require specialized knowledge and experience, making professional assistance invaluable.
- Time Constraints: Students juggling multiple courses and commitments may struggle to allocate sufficient time to thoroughly understand and complete Regression Analysis assignments, making timely help from experts essential.
- Access to Expertise: Online platforms provide students with access to a pool of experienced Regression Analysis experts who can offer personalized assistance tailored to their specific needs, ensuring a deeper understanding of concepts.
- Academic Performance: As Regression Analysis assignments often carry significant weight in overall grades, seeking online help can significantly improve academic performance and enhance prospects for future career opportunities.
A Winning Choice: The Benefits of Opting for Our Regression Analysis Assignment Help
Choosing our Regression Analysis assignment help service ensures a winning edge in your academic journey. With our team of seasoned regression analysis assignment experts at EconomicsAssignmentHelp.com, you gain access to tailored assistance that simplifies complex concepts and enhances your understanding. Our service provides comprehensive help with regression analysis assignments, guiding you through data interpretation, model building, and result analysis with precision. By opting for our assistance, you save time, alleviate stress, and boost your confidence in tackling regression tasks effectively.
If you are wondering who will do my Regression Analysis assignment? Trust us for top-notch economics assignment help that propels your academic success and empowers you to excel in your studies. Our live chat support ensures instant clarification of doubts, while our commitment to plagiarism-free work guarantees originality. Additionally, we offer free revisions to ensure your satisfaction. Delve into complex economics concepts with ease, backed by real-world case studies and fundamental economic theories tailored to your needs.
- Expert Guidance: Access tailored assistance from seasoned regression analysis experts, ensuring accurate interpretation of results and thorough understanding of complex statistical concepts.
- Time Efficiency: Save valuable time by leveraging our expertise to navigate through regression analysis tasks efficiently, allowing you to focus on other academic or personal commitments.
- Improved Grades: Receive high-quality solutions that adhere to academic standards, leading to improved performance and grades in regression analysis assignments and coursework.
- Stress Reduction: Alleviate the stress and pressure associated with challenging regression analysis tasks, knowing that you have reliable support and guidance every step of the way.
- Enhanced Confidence: Gain confidence in your regression analysis skills and abilities through personalized assistance and expert feedback, empowering you to tackle future assignments with assurance.
How to Get Our Regression Analysis Assignment Help Service?
To access our esteemed Regression Analysis Assignment Help service, simply follow these straightforward steps. First, submit your assignment details and requirements to economicsassignmenthelp.com. Our team of dedicated regression analysis assignment experts will review your task and provide a competitive quote. Once you approve the quote, make a secure payment through our platform. Sit back and relax as our experts work diligently on your assignment.
We'll notify you once the solution is ready for download. With our seamless process, obtaining top-notch economics assignment help has never been easier. If you are wondering who will do my Regression Analysis assignment? Trust us for expert guidance that ensures your academic success in regression analysis. Get the assistance you need with help with regression analysis assignment.
- Submit Your Assignment Details: Provide us with the specifics of your regression analysis assignment by filling out our online form on economicsassignmenthelp.com.
- Receive a Competitive Quote: Our team of econometrics assignment help experts will review your requirements and promptly provide you with a competitive quote for our services.
- Approve the Quote: Once you are satisfied with the quote, approve it to proceed with our service. Secure payment can be made through our platform.
- Sit Back and Relax: After approving the quote and making payment, our experts will diligently work on your assignment to deliver high-quality solutions.
- Download Your Solution: Once your assignment is completed, we'll notify you. Download your solution from our platform and feel confident in your regression analysis assignment submission.
Our Regression Analysis Assignment Help Service Covering Multiple Topics
Experience comprehensive Regression Analysis Assignment Help that spans a multitude of topics with economicsassignmenthelp.com. If you are wondering who will do my Regression Analysis assignment? Our team of regression analysis assignment experts is adept at providing tailored assistance across various subjects, ensuring that your specific academic needs are met. Whether you require help with regression analysis assignments related to simple linear regression, multiple regression, logistic regression, or any other variant, we have the expertise to guide you through.
Trust us for professional economics assignment help that covers a wide range of regression analysis topics, empowering you to excel in your coursework and achieve academic success. Our economics tutors specialize in social science and international economics, offering support with predictor variables and term papers. Let us assist you in mastering multiple linear regression and other essential concepts within the realm of regression analysis.
- Simple Linear Regression: Explore the foundational concepts of regression analysis by understanding the relationship between one independent and one dependent variable.
- Multiple Regression Analysis: Delve into the complexities of modeling relationships between multiple independent variables and one dependent variable.
- Logistic Regression: Learn how to analyze categorical outcomes by estimating the probability of a binary outcome using regression techniques.
- Time Series Analysis: Gain insights into analyzing time-dependent data to uncover patterns, trends, and forecast future values using regression methods.
- Panel Data Regression: Explore advanced regression techniques for analyzing data collected over time from multiple individuals, organizations, or regions, accounting for individual and time effects.
Diving Deep into Our Educational Regression Analysis Assignment Blogs
Embark on an enlightening journey through our regression analysis assignment help blogs, designed to demystify complex statistical concepts. Whether you're seeking help with regression analysis assignment or aiming to enhance your understanding, our curated content offers invaluable insights. Explore practical examples, step-by-step guides, and expert tips to master the nuances of regression analysis. Uncover methodologies, applications, and common pitfalls, all tailored to support your academic or professional endeavors. Let our regression analysis assignment helper resources empower your learning and elevate your analytical skills. Start exploring today!
Testimonials on Our Regression Analysis Assignment Help
Delve into firsthand accounts of students who found success with our regression analysis assignment help. From navigating complex datasets to interpreting results, our tailored assistance ensures clarity and comprehension. Students commend our help with regression analysis assignment for its depth of understanding and personalized approach. With the guidance of our experienced regression analysis assignment helper, they've conquered challenges and achieved academic excellence. Their testimonials highlight the effectiveness of our support in mastering regression analysis concepts and techniques. Join the ranks of satisfied students and experience transformative learning with us.
Rely on Our Seasoned Regression Analysis Assignment Helpers for Guaranteed Achievement
Count on our seasoned regression analysis assignment helpers to ensure your academic success. Our team is dedicated to providing comprehensive help with regression analysis assignments, guiding you through every step of the process. Benefit from personalized support and expert insights tailored to your needs. With our experienced regression analysis assignment helper by your side, you can tackle complex topics with confidence and achieve guaranteed results. Enhance your understanding of regression analysis concepts and excel in your coursework. Trust our proven track record of empowering students to excel in their academic endeavors.
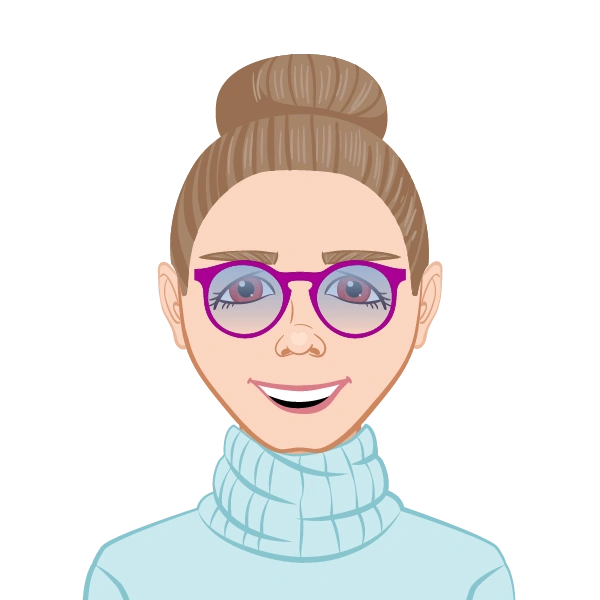
Kate Mistry
Ph.D. in Economics
🇬🇧 United Kingdom
Kate Mistry, PhD in Economics from the University of North Dakota, is an expert in Econometrics with 8 years of experience. She has successfully completed over 950 assignments, specializing in regression analysis, causal modeling, and data interpretation. Her expertise helps organizations leverage statistical insights to make informed economic decisions and optimize strategies effectively.
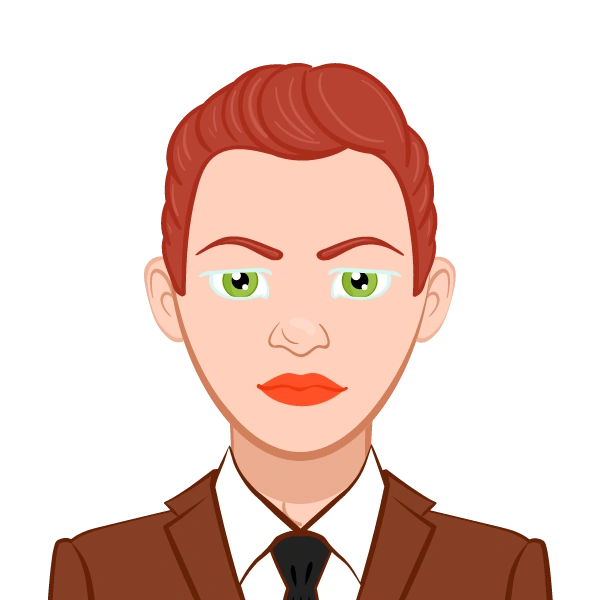
Ryan Miller
Ph.D. in Economics
🇺🇸 United States
Ryan Miller, PhD in Economics from the University of Wyoming, is an expert in Econometrics with 8 years of experience. He has completed over 850 assignments, focusing on regression analysis, time series forecasting, and statistical modeling. His analytical skills enable organizations to derive meaningful insights from data and enhance their decision-making processes effectively.
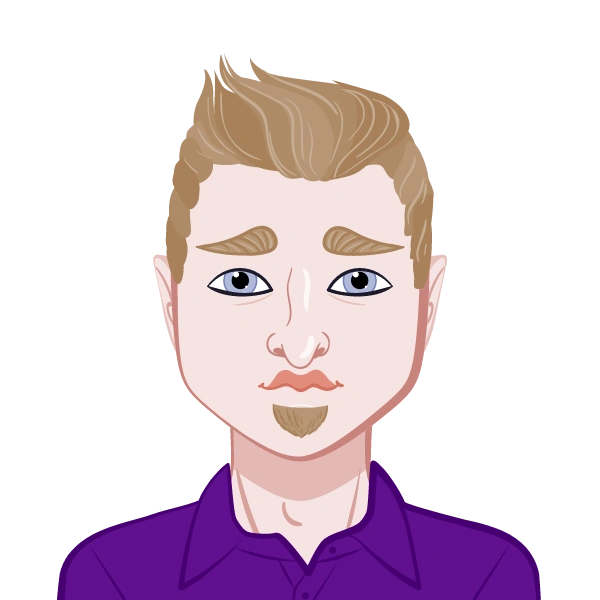
Tore Berglund
Ph.D. in Economics
🇺🇸 United States
Tore Berglund, PhD in Economics from the University of Idaho, is an expert in Econometrics with 8 years of experience. I have successfully completed over 750 assignments, specializing in regression analysis, causal inference, and data modeling. My expertise empowers organizations to extract valuable insights from data and make informed economic decisions effectively.
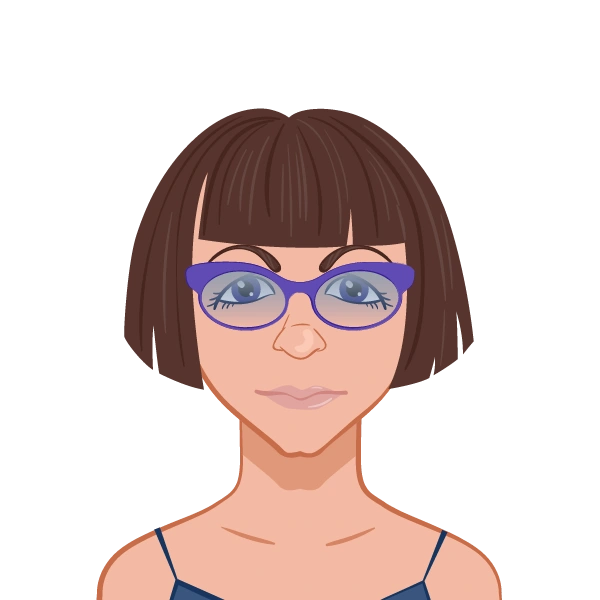
Natalia Lundin
Ph.D. in Economics
🇨🇭 Switzerland
Natalia Lundin, PhD in Economics from the University of Fribourg, is an expert in Econometrics with 8 years of experience. I have completed over 700 assignments, specializing in regression analysis, time series forecasting, and data interpretation. My analytical skills enable organizations to leverage data effectively for informed decision-making and strategic planning.
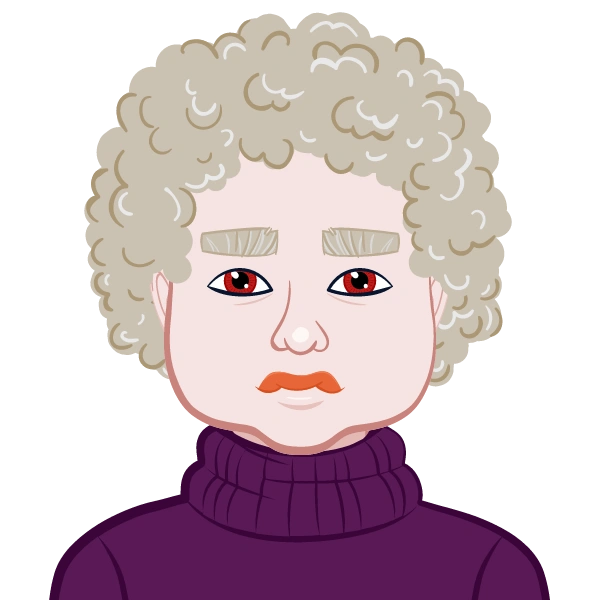
Billy A. Laroche
Ph.D. in Economics
🇺🇸 United States
Billy A. Laroche, PhD in Economics from the University of Maine, is an expert in Econometrics with 8 years of experience. I have successfully completed over 650 assignments, focusing on regression analysis, time series modeling, and causal inference. My expertise helps organizations derive actionable insights from data and make informed economic decisions.
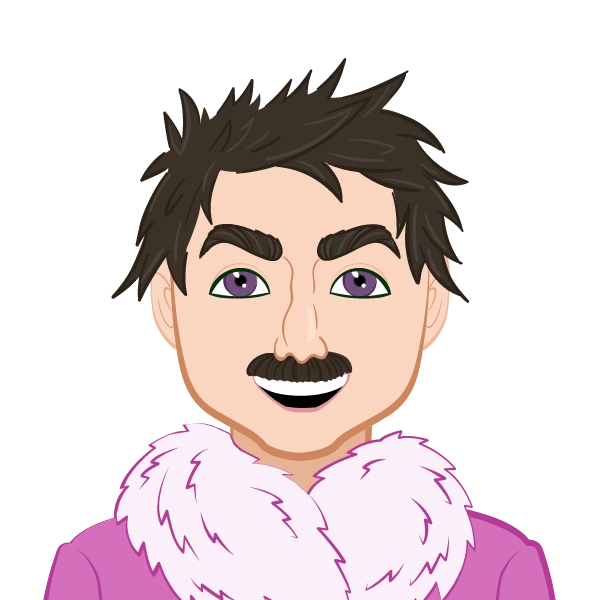
Steven P. Weinberger
Ph.D. in Economics
🇺🇸 United States
Steven P. Weinberger, PhD in Economics from the University of Montana, is an expert in Business Economics with 8 years of experience. I have successfully completed over 550 assignments, specializing in economic analysis, market strategies, and policy evaluation. My insights empower organizations to navigate complex economic challenges and make data-driven decisions effectively.
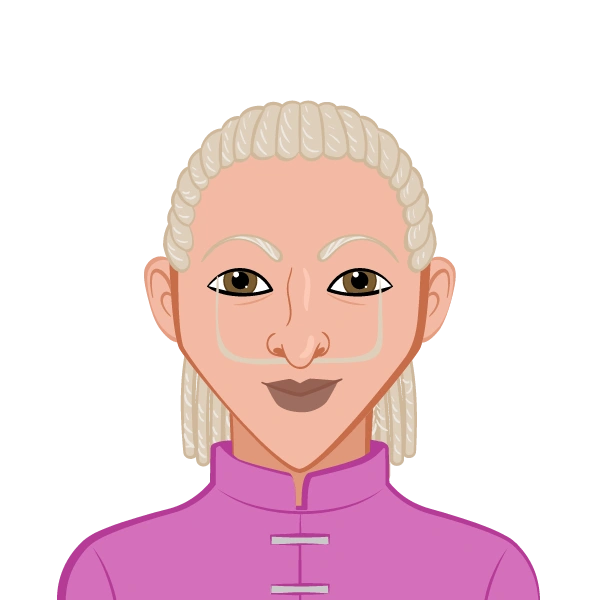
Riley Goossens
Ph.D. in Economics
🇦🇺 Australia
Riley Goossens, PhD in Economics from the University of Wollongong, is an expert in Business Economics with 12 years of experience. I have successfully completed over 400 assignments, specializing in economic analysis, market strategies, and policy evaluation. My insights empower organizations to navigate complex economic challenges and enhance their decision-making processes effectively.

Olivia Dennys
Ph.D. in Economics
🇦🇺 Australia
Olivia Dennys, PhD in Economics from the University of Newcastle, is an expert in Business Economics with 12 years of experience. She has successfully completed over 400 assignments, focusing on economic analysis, market strategies, and policy implications. Her insights help organizations enhance performance and navigate complex economic challenges effectively.
Dr. Sienna Callahan
Ph.D. in Economics
🇬🇧 United Kingdom
Dr. Sienna Callahan, PhD in Economics from the University of Amsterdam, Netherlands, brings over 9 years of experience to our team. Having completed over 900 Rational Expectations Theory assignments, Sienna is known for her comprehensive understanding of economic principles and her dedication to assisting students in achieving their academic goals.
Dr. Isla Dempsey
Ph.D. in Economics
🇬🇧 United Kingdom
Dr. Isla Dempsey, a seasoned economist, holds a PhD from the University of Edinburgh, Edinburgh, UK. With over 8 years of experience, Isla has successfully completed more than 800 Rational Expectations Theory assignments. Isla's commitment to academic excellence and her ability to simplify complex economic concepts make her a valuable resource for students seeking help with their assignments.
Dr. Freya Langley
Ph.D. in Economics
🇺🇸 United States
Dr. Freya Langley, a distinguished scholar with a PhD from Columbia University, New York, USA, brings over 7 years of experience to our team. Having completed over 700 Rational Expectations Theory assignments, Freya's profound understanding of economic theories and practical applications enables her to offer insightful guidance and meticulous solutions to students seeking assistance.
Dr. Imogen Sullivan
Ph.D. in Economics
🇦🇪 United Arab Emirates
Dr. Imogen Sullivan holds a PhD in Economics from the University of Pennsylvania, Philadelphia, USA. With over 6 years of experience, she has successfully completed more than 600 Rational Expectations Theory assignments. Imogen's expertise lies in providing in-depth analysis and precise solutions to complex economic problems, ensuring students achieve academic excellence in their assignments.
Dr. Olive Wong
Ph.D. in Economics
🇺🇸 United States
Dr. Olive Wong, with a Ph.D. in Economics from Tokyo University, Dr. Wong is a prominent figure in the field, boasting over 8 years of experience. Having completed over 800 Economic Forecasting Assignments, she is revered for her deep understanding of global economic trends and her ability to generate accurate forecasts that drive informed decision-making for businesses and organizations worldwide.
Dr. Eliza Thomas
Ph.D. in Economics
🇨🇦 Canada
Dr. Eliza Thomas, distinguished alumna of McGill University, Dr. Thomas possesses an unparalleled expertise in Economics, honed over 7 years of experience. Having successfully delivered over 700 Economic Forecasting Assignments, she is recognized for her strategic insights and data-driven approach, consistently exceeding client expectations with her thorough analyses and actionable recommendations.
Dr. Adelaide Knight
Ph.D. in Economics
🇦🇺 Australia
Dr. Adelaide Knight, holding a Ph.D. in Economics from ETH Zurich, Dr. Knight is a seasoned expert with over 6 years of experience in the field. With a remarkable track record of completing over 600 Economic Forecasting Assignments, she is celebrated for her comprehensive understanding of economic theories and her ability to apply them to real-world scenarios.
Dr. Imogen Armstrong
Ph.D. in Economics
🇺🇸 United States
Dr. Imogen Armstrong, with a Ph.D. in Economics from the University of Melbourne, Dr. Armstrong brings over 5 years of experience to the table. Having completed over 500 Economic Forecasting Assignments, she is renowned for her innovative approaches and meticulous analysis, ensuring accurate predictions and insightful insights for clients worldwide.
Prof. Jake Burns
Ph.D. in Economics
🇨🇦 Canada
Professor Jake Burns, a graduate of the esteemed University of British Columbia, Canada, holds a Ph.D. in Economics. With a decade of expertise, he excels in Time Series Analysis, employing advanced methodologies to deliver precise insights.
Prof. Finlay Anderson
Ph.D. in Economics
🇦🇺 Australia
Professor Finlay Anderson, a distinguished graduate of the University of Melbourne, Australia, brings over 12 years of invaluable expertise in Time Series Analysis. With a Ph.D. earned in the field, his profound knowledge enriches every aspect of his work.
Prof. Aidan Heath
Ph.D. in Economics
🇺🇸 United States
Professor Aidan Heath, a Carnegie Mellon University alumnus, holds a Ph.D. in Time Series Analysis. With 15 years of experience, his expertise spans advanced methodologies, including SARIMA modeling, ensuring insightful analyses and academic excellence.
Prof. Jay Murray
Ph.D. in Economics
🇬🇧 United Kingdom
Prof. Jay Murray, a distinguished graduate of the University of Aberdeen, United Kingdom, holds a Ph.D. in Time Series Analysis. With 18 years of experience, his expertise in advanced methodologies such as ARIMA modeling is unparalleled.
Related Topics
Frequently Asked Questions (FAQs)
Looking for answers regarding regression analysis assignment help? Explore our FAQs for comprehensive information on our services. Discover how our help with regression analysis assignment can benefit your academic journey. Find solutions to common queries about deadlines, pricing, expertise of our regression analysis assignment helper, and more. Whether you're a student seeking assistance or a professional aiming to enhance your skills, our FAQs provide clarity and guidance. Get started today and unlock the support you need to succeed in your regression analysis assignments.